COMBINE immerses graduate students in interdisciplinary research and training that integrates quantitative modeling methods from physics and mathematics with data processing, analysis, and visualization tools from computer science to gain deeper insights into the structural and dynamical principles governing living systems. Participants utilize a network-based, data-driven approach, focusing on how interaction patterns can give insights into complex biological phenomena. COMBINE prepares students to become experts in the process of transforming raw biological data into useful information from which new biological insights can be inferred, positioning them to pursue a range of Science, Technology, Engineering, and Mathematics (STEM) careers at the nexus of the computer, physical, and life sciences.
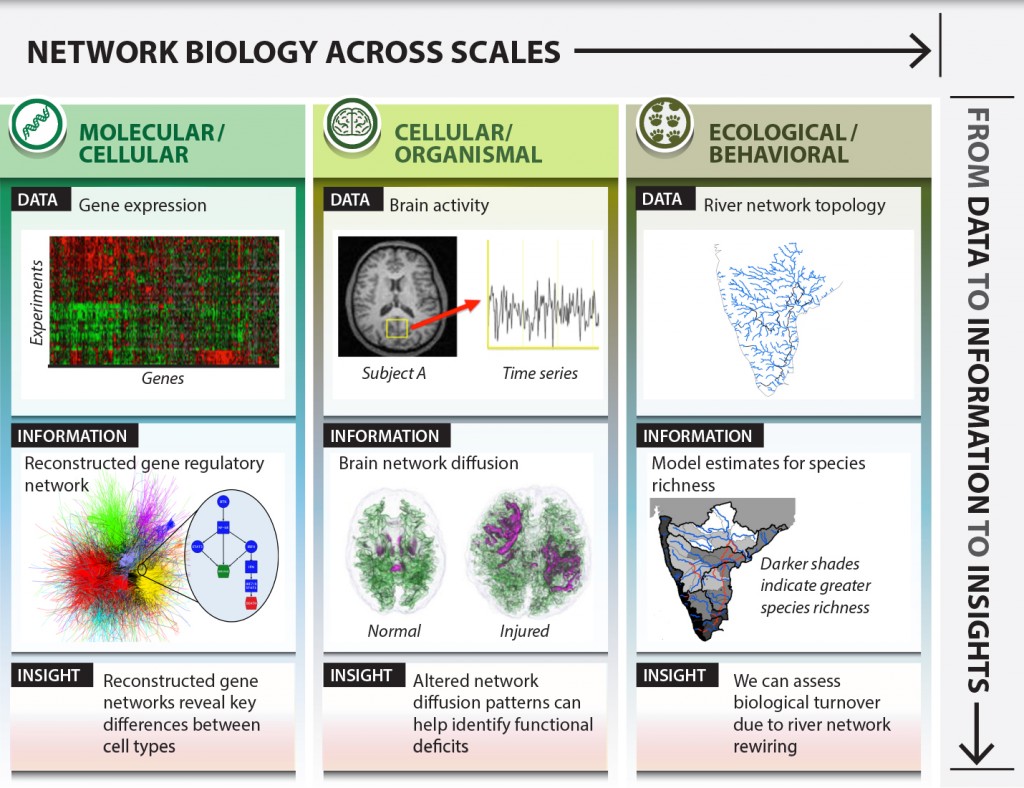
Participants receive training in four areas of network analysis: quantitative metrics for biological networks; mechanistic models of biological networks; network statistics and machine learning for biological applications; and visualization techniques for large, complex biological data sets. This training provides the foundation for research in one or more of the following areas: biomolecular, neuronal and/or ecological/behavioral networks.
Take a peek at our program evaluation outcomes and get more details about it in our PLOS One paper.
COMBINE is supported by the University of Maryland and through NSF award number 1632976.